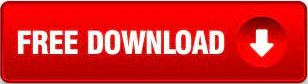

But given the many technical solutions to this problem, from sophisticated deidentification methods to highly secure cloud environments, this cannot be the only reason. Highly talented researchers who could make major contributions to medicine are diverted into solving trivial problems in other fields.Ī commonly cited reason for these barriers to access is the protection of patient privacy. Their performance cannot be adequately scrutinized, leading to failures of replication and erosion of trust 10. Algorithms are designed largely to serve the needs of the privileged 9. This has a variety of negative consequences. Access for everyone else is laborious, costly, time-consuming or just impossible, despite the fact that the creation of nearly all health data, whether from insurance premiums or research grants, is publicly funded. Instead, they are often controlled by a handful of researchers at well-resourced institutions or companies. Datasets meeting these two criteria are the ‘secret sauce’ of machine learning - more than just computing power, or individual genius - and underlie the unprecedented recent progress in translation, sentiment analysis, object and facial recognition, and other tasks 8 (Table 1).Įxisting health datasets seldom meet these two criteria.
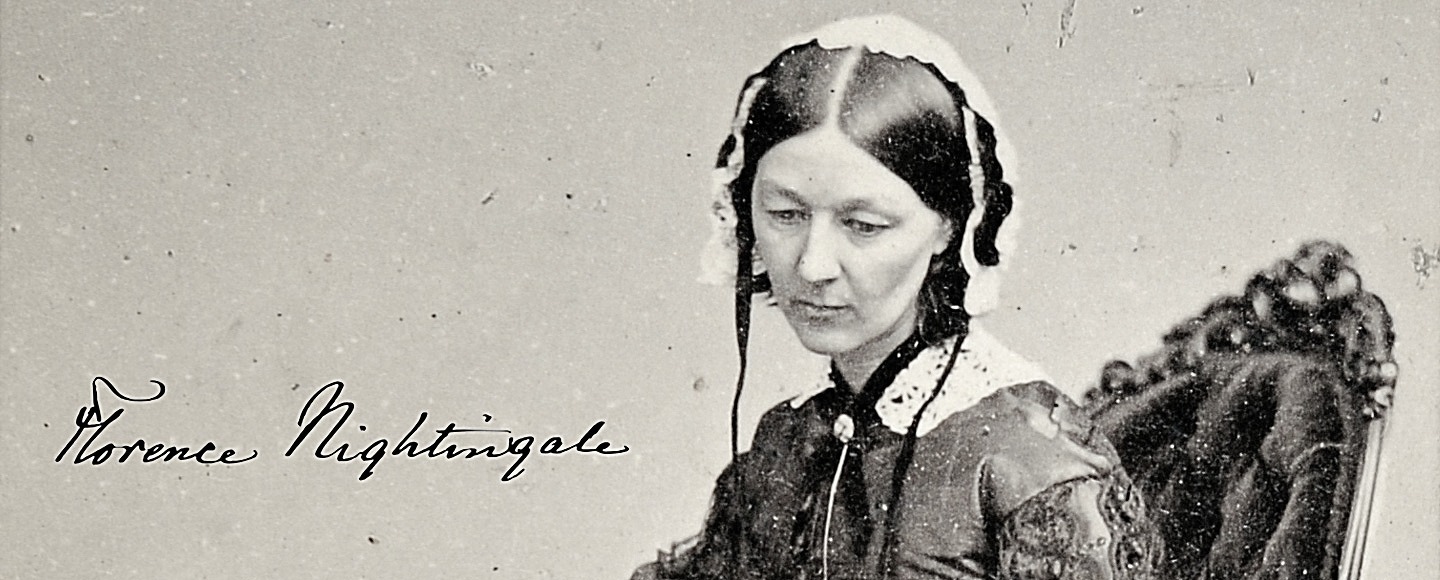
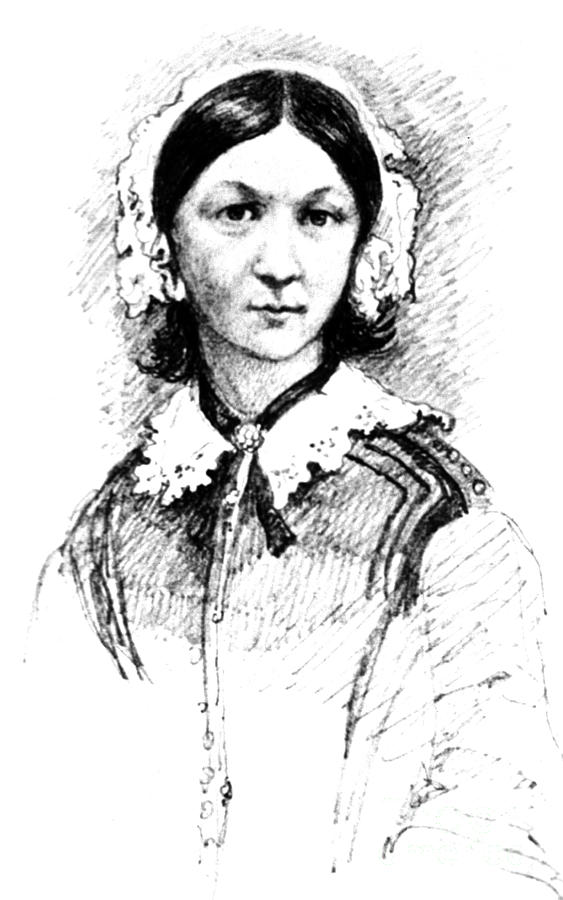
Second, the data must be curated around ‘common tasks’: important, field-defining problems on which a community of researchers can collaborate, compete and improve. Only then can good ideas thrive, on a level and just playing field. Instead, the data must be accessible at low cost, in terms of money and in terms of time. First, they must be open access: they cannot be monopolized by those who produce it, whether academics, non-profits or corporations. Instead, recent successes from other disciplines - genomics, computational biology, language modeling, and image recognition, to name a few - suggest that datasets must also possess two specific features. Solving medicine’s data bottleneck: Nightingale Open Science.Like any scientific field, medicine needs data to grow and thrive. MLA Mullainathan, Sendhil, and Ziad Obermeyer. “Solving Medicine’s Data Bottleneck: Nightingale Open Science.” Nature Medicine, vol. “Solving Medicine’s Data Bottleneck: Nightingale Open Science.” Nature Medicine 28, no. The problemĪ patient is rushed into the ER, unconscious and in cardiac arrest. What happened to cause the arrest? What immediate actions need to be taken? And what will happen to the patient? As the physician begins the resuscitation, she knows only that the patient’s heart has stopped-but nothing else. One of the only pieces of data available to the emergency physician in this situation is the electrocardiogram (ECG), which measures the electrical activity of the heart. Physicians use this to determine which immediate actions are needed: most importantly, does the patient need to be shocked (cardioverted), or need some critical medication to restart the heart.

This rich signal might also contain other clues: about why the heart stopped, what physicians can do in the ER to give the patient the best possible chance of surviving, and the likelihood that a patient who survives will have a normal life, without profound physical or neurological impairments. Over the past 10 years, the National Taiwan University Hospital (NTUH) has captured and stored ECG waveforms from all adult (over 20 years old) Emergency Department (ED) patients in cardiac arrest: those brought in by ambulance in cardiac arrest, and those experiencing cardiac arrest in the ED or the waiting room. Research staff would identify patients in the ED with cardiac arrest, and physicians would then review the medical records and enter the patient into a ‘registry’ dataset, using an Utstein-style reporting template. The team would also monitor the patient’s course in the ED and the hospital, to enter a variety of other outcome data: the patient’s survival and neurological function, and what the eventual cause of the arrest was determined to be, by the doctors who cared for the patient in the hospital. Our partnersĮstablished in 1895, National Taiwan University Hospital (NTUH) is a massive, 2,300-bed hospital located in Taipei. It’s the largest hospital in Taiwan, with 1,300 full-time physicians. Its busy emergency department sees approximately 100,000 patients every year. It’s the leading hospital for cardiovascular treatment in Taiwan, treating around 3,400 cardiac catheterization cases every year. This dataset was conceived of and created by Dr. Chien-Hua Huang, Chairman and clinical professor in the Department of Emergency Medicine at NTUH, with invaluable assistance from Dr.
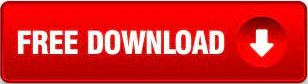